DATA SCIENCE WITH PYTHON & R
PYTHON + R + MYSQL + SQL + POWERBI + BIG DATA
Discover endless possibilities for growth and success in the IT industry with Insta Dot Analytics. Our career-focused programs and personalized support prepare you to excel in high-demand fields such as cybersecurity, cloud computing, data science, and more.
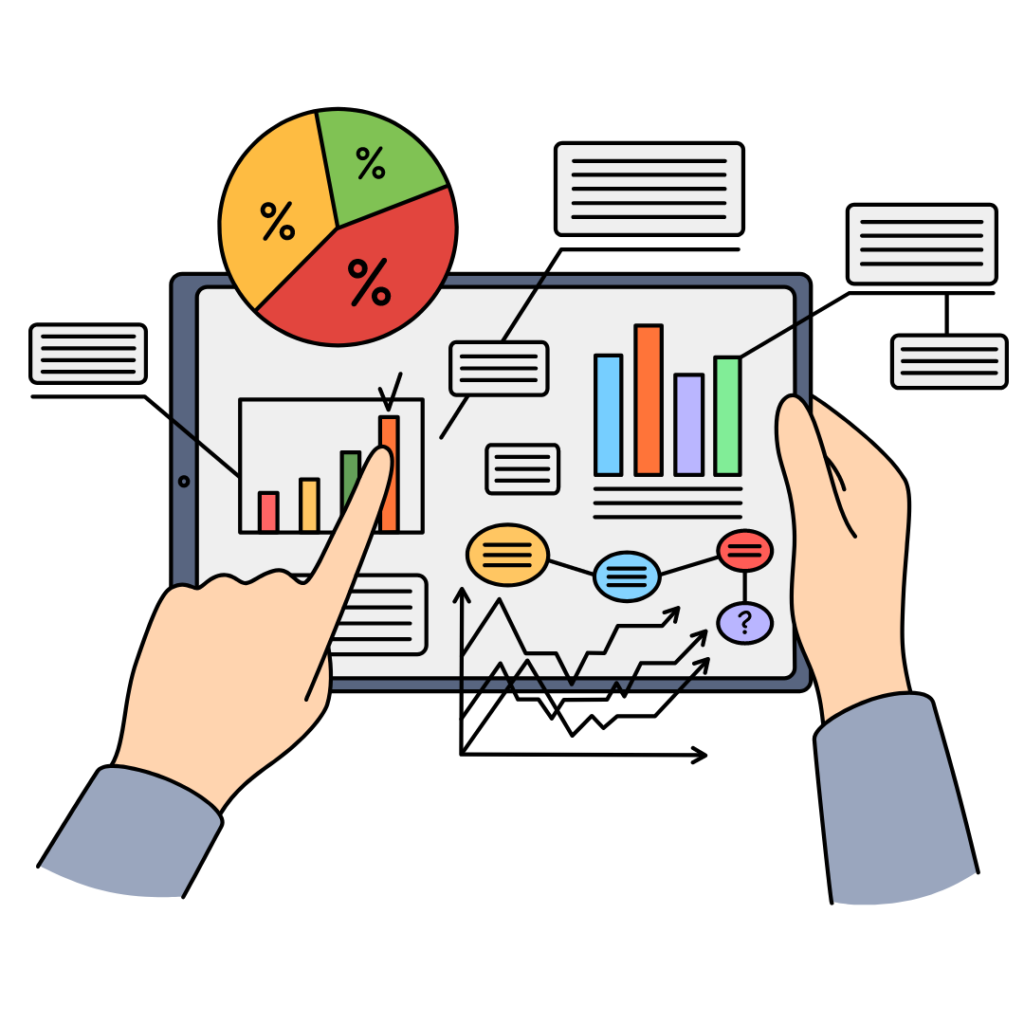
GETTING STARTED
- INTRODUCTION
- COURSE OVERVIEW
- DIFFERENT SECTORS USING DATA SCIENCE
- DATA ANALYTICS PROCESS
- WHY DATA SCIENCE
- WHO’S USING DATA ANALYTICS?
- INSTALLATION
- INSTALLING DEPENDENCIES
- INSTALLING SOFTWARES
DATA SCIENCE WITH R
- R PROGRAMMING
- INTRODUCTION
- INSTALLATION OF LIBRARIES
- CONSTANTS AND VARIABLES
- OPERATORS
- DATA TYPE
- CONDITIONAL STATEMENT
- R - FUNCTIONS
- CREATE DATA FRAMES
- EDA USING R
- READING DIFFERENT FILE FORMATS
- STATICAL ANALYSIS
- GGPLOT2 LIBRARY
PYTHON PROGRAMMING
- INTRODUCTION
- ENVIRONMENT SETUP
- INTRODUCTION TO JUPYTER NOTEBOOK
- PSEUDOCODE
- VARIABLES
- COMMENTS
- OPERATORS
- DATA TYPES
- DATA CONVERSION
- CONDITIONAL STATEMENTS
- ADVANCE DATA TYPES
- FUNCTIONS
- METHODS
- CLASS AND OBJECTS
- MODULES AND PACKAGES
- INBUILT MODULES OR LIBRARIES
- NUMPY
- DATAFRAME MANIPULATION – PANDAS
- SCIPY
- DATA VISUALIZATION – MATPLOTLIB OR SEABORN
- EDA – BASIC STATISTICS METHODS IN PYTHON
- SCIKIT LEARN BASICS
SQL
- BASIC MYSQL
- INTRODUCTION TO SQL
- DDL AND DML STATEMENT
- SELECT STATEMENT
- AGGREGATE FUNCTIONS
- WHERE, ORDER BY, DISTINCT, GROUP BY
- LIKE, AND & OR CLAUSE
- UPDATE & DELET QUERY
- ADVANCED MYSQL
- JOINS
- UNION, UNION ALL, INTERSECT
- USING VIEWS & INDEXES
STATISTICS AND PROBABILITY
- ABOUT DATA
- DATA DEFINITION
- RAW AND PROCESSED DATA
- DATA TYPES (NOIR)
- DESCRIPTIVE STATS
- MEASURES OF CENTRAL TENDENCY
- MEASURE OF DISPERSION
- MEASURE OF ASSOCIATION
- PROBABILITY
- BASIC TERMINOLOGY
- RULES AND EVENTS
- CONDITIONAL PROBABILITY AND BAYES THEOREM
MACHINE LEARNING WITH PYTHON
- SUPERVISED LEARNING
- BASIC TERMINOLOGY
- ML FUNDAMENTALS:
- ML MODELLING FLOW
- PARAMETRIC AND NON-PARAMETRIC ML ALGORITHM
- TYPES OF ML
- PERFORMANCE MEASURES
- BIAS-VARIANCE TRADE-OFF
- OVERFITTING AND UNDERFITTING
- LINEAR REGRESSION: LINEAR REGRESSION WITH OLS
- LINEAR REGRESSION WITH SGD
- EVALUATING MODEL PARAMETERS
- L1 AND L2 REGULARIZATION
- MEASURING PERFORMANCE METRICS
- LOGISTIC REGRESSION
- LOGISTIC REGRESSION MLE
- LOGISTIC REGRESSION WITH SGD
- EVALUATING MODEL PERFORMANCE
- UNSUPERVISED LEARNING
- PRINCIPAL COMPONENT ANALYSIS
- INTRO TO DIMENSIONALITY REDUCTION
WHAT IS PCA?
BIG DATA AND HADOOP
- HADOOP FRAMEWORK
- LINUX COMMANDS & SHELL
- CREATING AND EXECUTING LINUX SCRIPT
- INTRODUCTION TO BIG DATA
- HADOOP ECO - SYSTEM
- HDFS ARCHITECTURE
- MAP - REDUCE BASICS
- HIVE
- PIG
- SQOOP & FLUME - DATA INGESTION
- OOZIE
- HBASE
DATA HANDLING WITH POWERBI
- POWERBI INTRODUCTION
- VISUALIZATION WITH BI
- DATA ANALYSIS EXPRESSIONS
OUR FUN AND ENGAGING CASE STUDIES INCLUDE:
- STATISTICAL AND DATA ANALYSIS CASE STUDIES:
- PREDICTING THE US 2020 ELECTION USING MULTIPLE POLLING DATASETS
- PREDICTING DIABETES CASES FROM HEALTH DATA
- MARKET BASKET ANALYSIS USING THE APRIORI ALGORITHM
- PREDICTING THE FOOTBALL/ SOCCER WORLD CUP
- COVID ANALYSIS AND CREATING AMAZING FLOURISH VISUALISATIONS (BARCHART RACE)
- PREDICTIVE MODELING AND CLASSIFIERS CASE STUDIES:
- PREDICTING AIRBNB PRICES
- DETECTING CREDIT CARD FRAUD